Benchmark
Following the existing vision-language pre-training models, we employ a dual-encoder architecture for vision-language representation learning. Since the encoders of visual and textual modalities are decoupled, we explore different encoder architectures. Benchmark code is available at Mindspore and Pytorch project.
Model | Embedding dimension | Image encoder | Similarity | # vis Token | Checkpoints |
---|---|---|---|---|---|
\(CLIP_{ViT-B}\) | 512 | ViT-B/32 | Global | / | Mindspore: Google Drive / Baidu Yunpan
Pytorch: Google Drive / Baidu Yunpan |
\(FILIP_{ViT-B}\) | 256 | ViT-B/32 | Token-wise | / | Mindspore: Google Drive / Baidu Yunpan
Pytorch: Google Drive / Baidu Yunpan |
\(Wukong_{ViT-B}\) | 256 | ViT-B/32 | Token-wise | 12 | Mindspore: Google Drive / Baidu Yunpan
Pytorch: Google Drive / Baidu Yunpan |
\(CLIP_{ViT-L}\) | 768 | ViT-L/14 | Global | / | Mindspore: Google Drive / Baidu Yunpan
Pytorch: Google Drive / Baidu Yunpan |
\(FILIP_{ViT-L}\) | 256 | ViT-L/14 | Token-wise | / | Mindspore: Google Drive / Baidu Yunpan
Pytorch: Google Drive / Baidu Yunpan |
\(Wukong_{ViT-L}\) | 256 | ViT-L/14 | Token-wise | 24 | Mindspore: Google Drive / Baidu Yunpan
Pytorch: Google Drive / Baidu Yunpan |
We evaluate our models on several tasks, for zero-shot classification task:
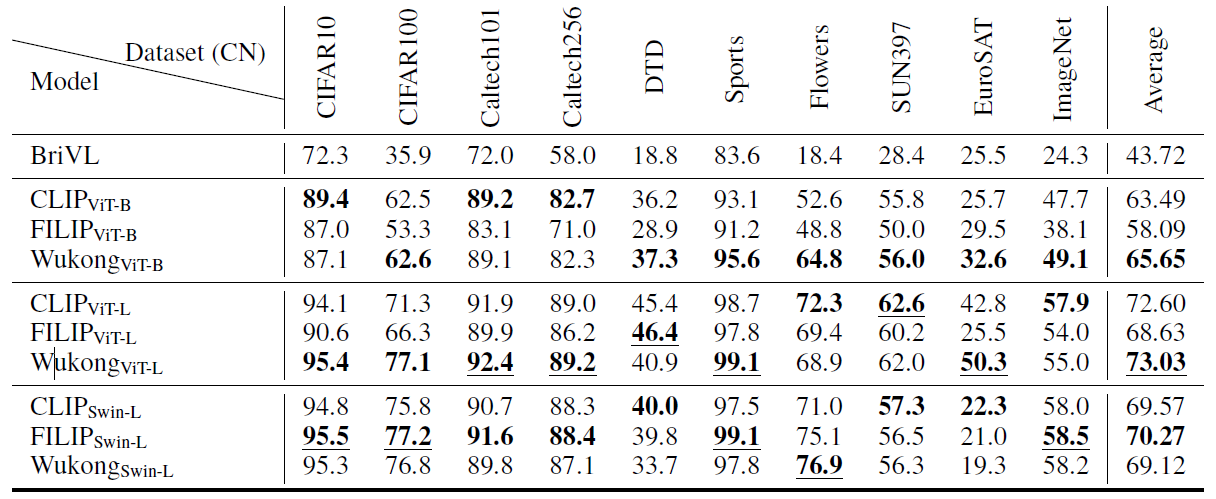
For zero-shot image text retrieval task:
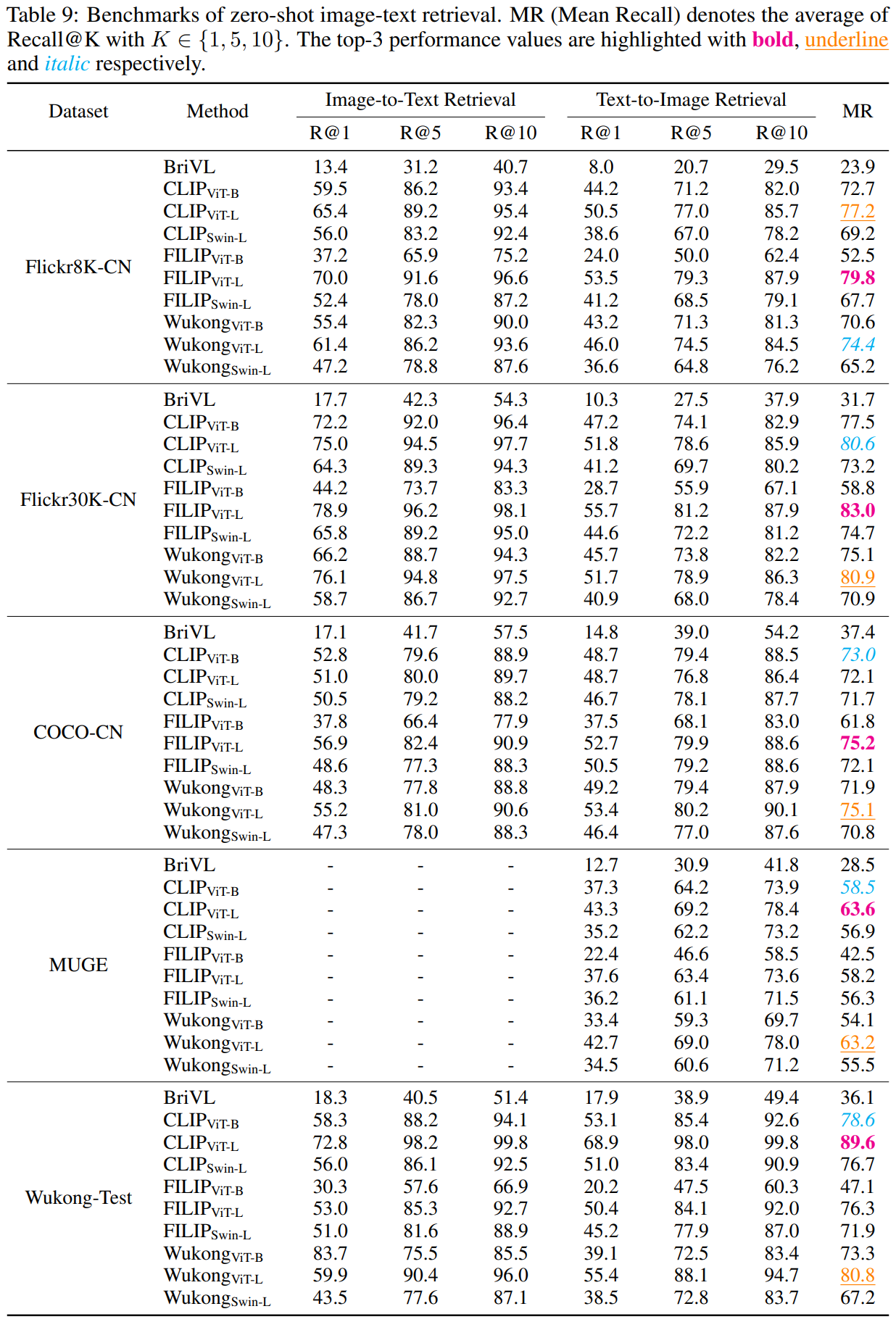
And for finetuned retrieval task:
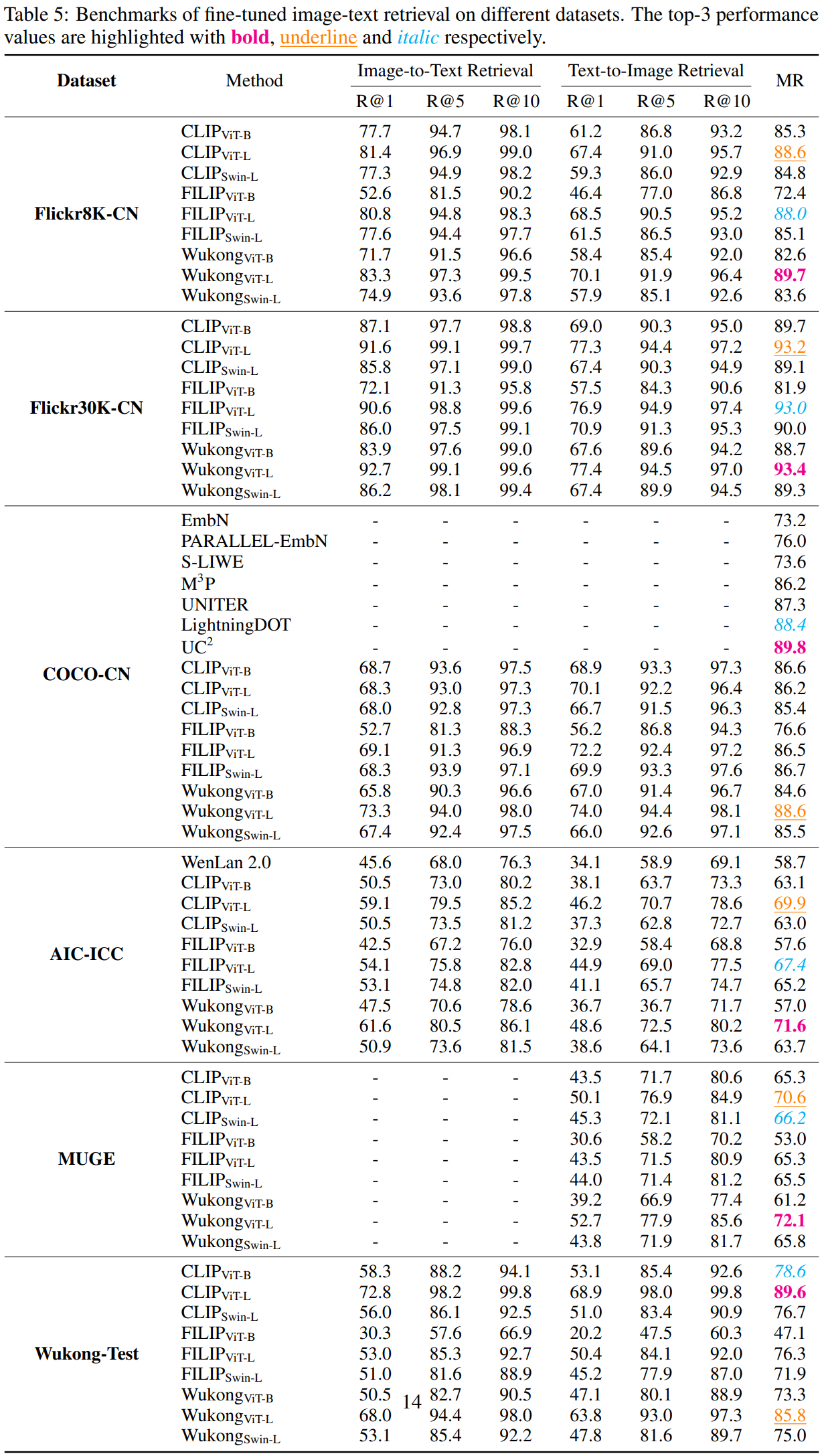
Below is some visualization examples of our models.
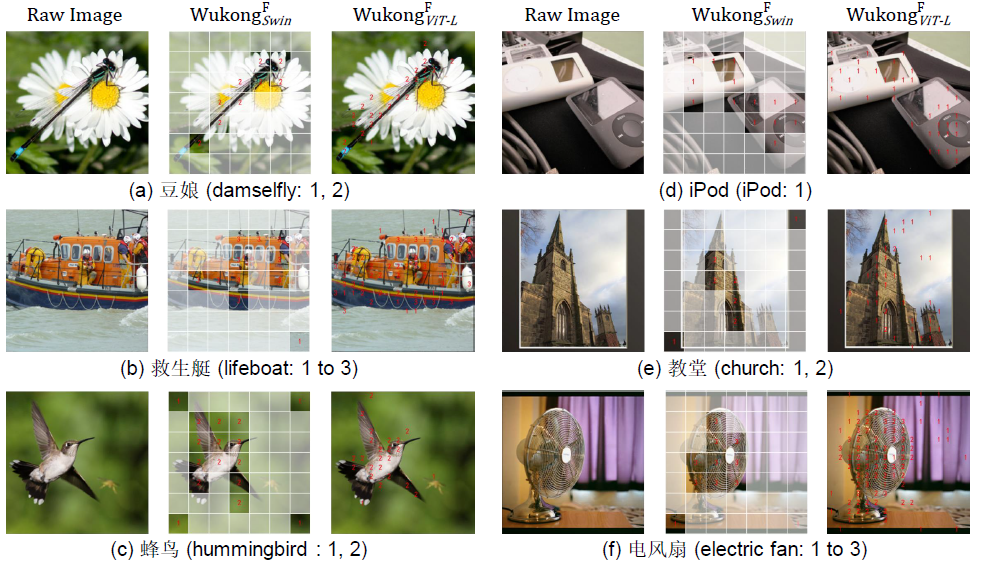